Use-Inspired Research
Coastal Oceanography
Improving the prediction and understanding of ocean phenomena through AI/ML methods, impacting our local communities and most vital industries.
Sea Turtle Conservation
In the Laguna Madre, the longest hypersaline lagoon in the United States, the passage of cold fronts can lower air temperature by more than 10°C in less than 24 hours. This can lead to a considerable decrease in water temperature. Some of these cold-water events result in large fish kills and sea turtle cold-stunning.
To mitigate the impact of these cold events, local agencies, private sector companies and other stakeholders (logos below) voluntarily interrupt activities such as fishing and navigation in the Laguna Madre. To manage these interruptions and mobilize resources, accurate predictions are very helpful.
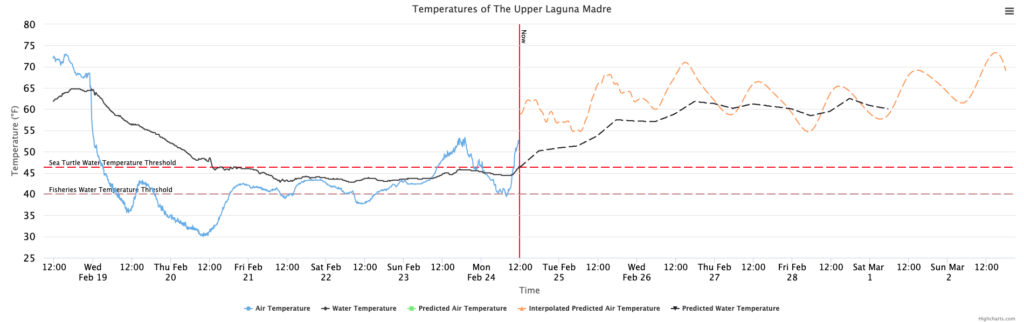
In collaboration with The Weather Company, students and faculty at Texas A&M — Corpus Christi have developed an AI operational prediction model (shallow neural net) to predict water temperatures in the Laguna Madre. This provides up to 120 hours lead time facilitating the decision process ahead of cold-stunning events.
The team provided predictions and guidance for the determination and adjustment of the start and stop of navigation interruptions during a February 2022 cold-stunning event.
In addition, our team continues to engage with stakeholders, including a tentative agreement from stakeholders such as the Texas Marine Cold-water Response Collaborative and Texas Parks and Wildlife to participate. Read more on this collaboration here.
Related Projects: Risk communication for Cold Water Temperature Prediction
Highlighted Publications and Releases
We have yet to add these publications. In the mean time explore our publications.
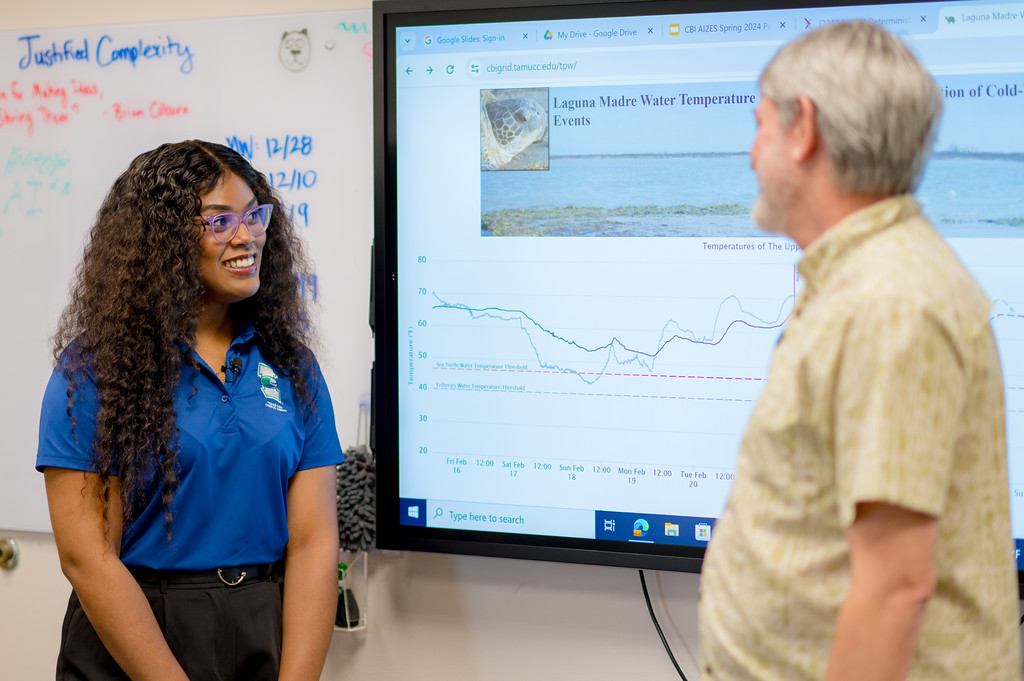
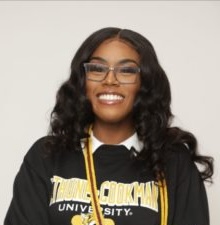
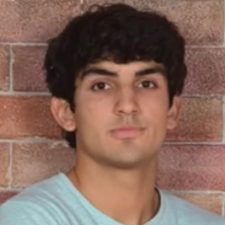
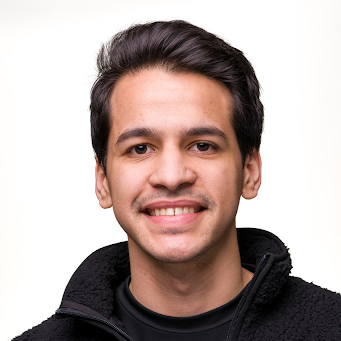
Coastal Inundation
Coastal inundations can have a major impact on beach management and safety. The frequency of flooding is increasing exponentially with relative sea level rise. This creates a need for more accurate models that can predict potential coastal inundation and alert beachgoers and officials of related hazards.
The team is working on the creation of an AI inundation model capable to predict where the water will reach on the beach. This will be an invaluable addition to models that predict water levels at tide gauges but without including the water runup on the beach. Models predicting inundations on an hourly basis will help counties and cities better manage their beaches.
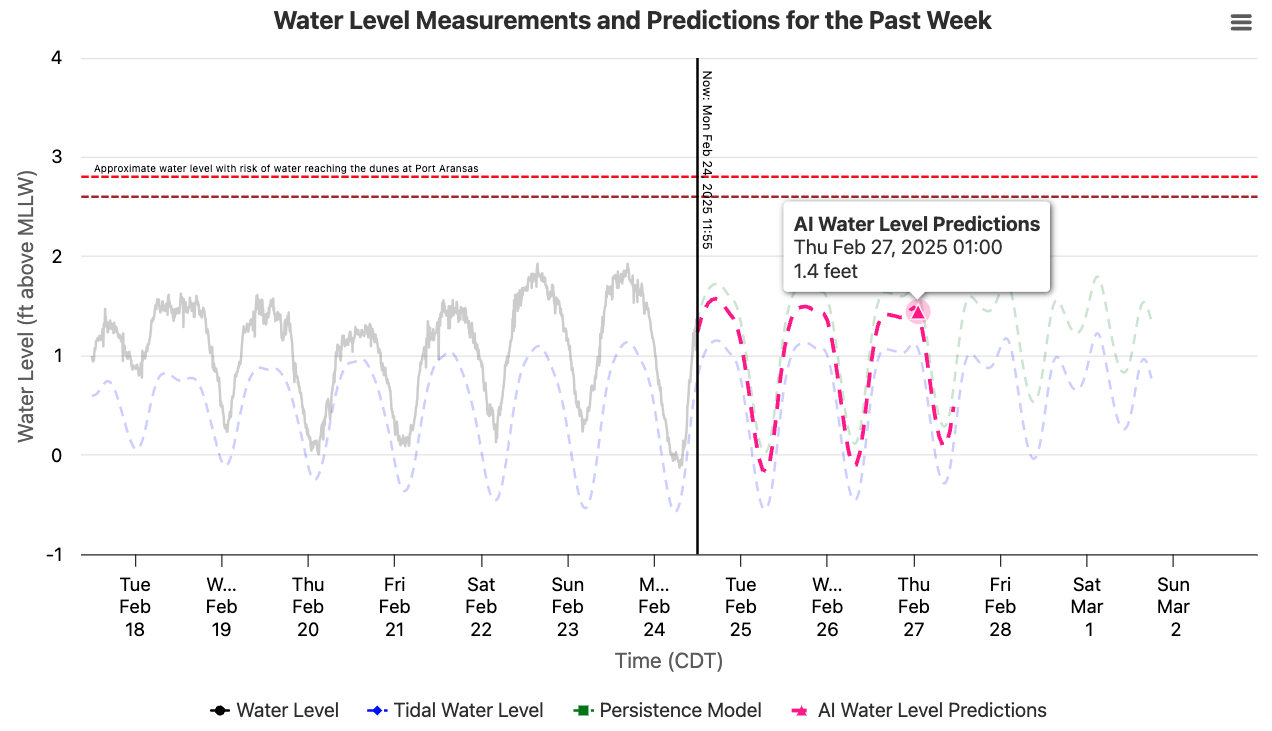
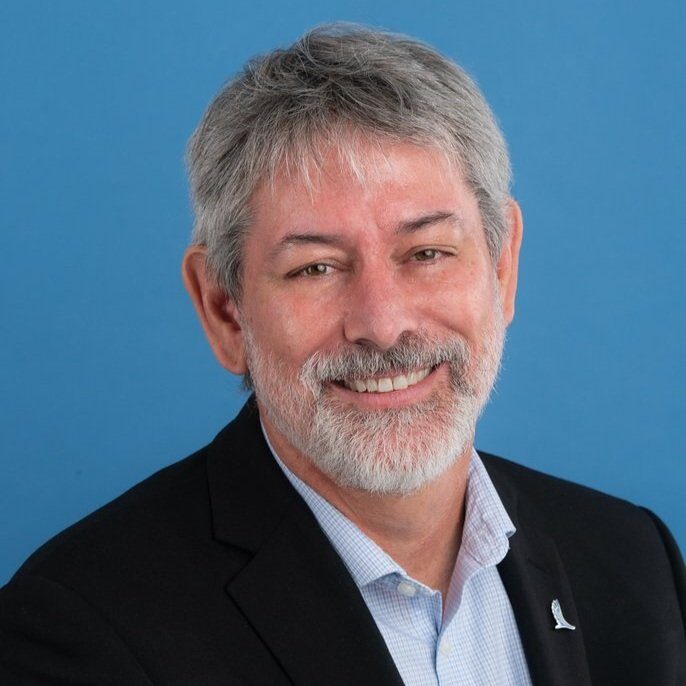
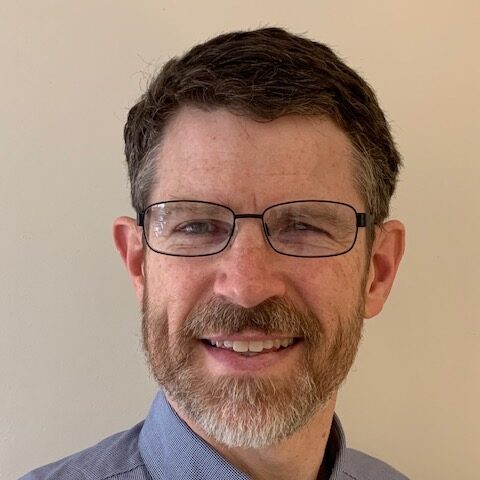
NSF AI2ES faculty and students have created a dataset of over 3,000 drone-based coastal images from 12 locations in Florida and Texas with labeled shoreline wet/dry line including elevations and Deep Learning predictions.
We installed an extended set of cameras, including a stereo camera, on a local pier to provide point cloud time series data on coastal shoreline.
A team of undergraduate students developed water level predictions visualizations for stakeholders and scientists (see right). These predictions are not only provided to stakeholders, such as the Nueces County Coastal Parks, but also available to the public online through Texas A&M – Corpus Chrisiti.
Highlighted Publications and Releases
We have yet to add these publications. In the mean time explore our publications.
Coastal Fog Predictions: FogNet
Coastal fog is a safety concern for transportation, particularly in a coastal city with frequent ship and plane travel. CBI NSF AI2ES team has developed a sophisticated deep learning model, known as FogNet, which combines satellite sea surface temperatures and numerical model outputs to predict the onset of fog with 9 to 24 hours advance warning.
The fog models are presently in their research stages, but NSF AI2ES collaborators including CBI, the National Center for Atmospheric Research (NCAR), and The Weather Company/IBM, are working together to create operational models that are high performing, cost-effective, and meet most of the expectations of future users.
Related Projects: Trustworthy XAI for FogNet with User Interviews
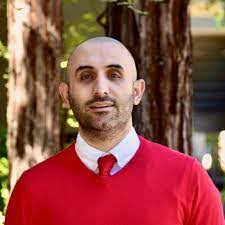
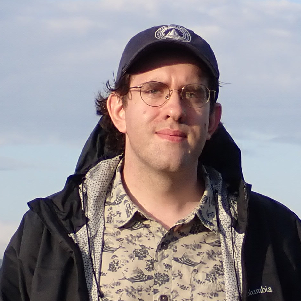
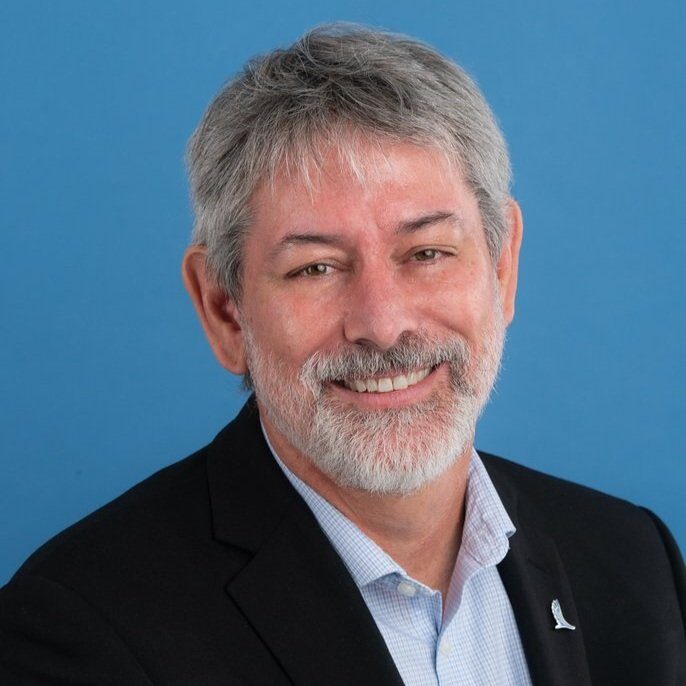
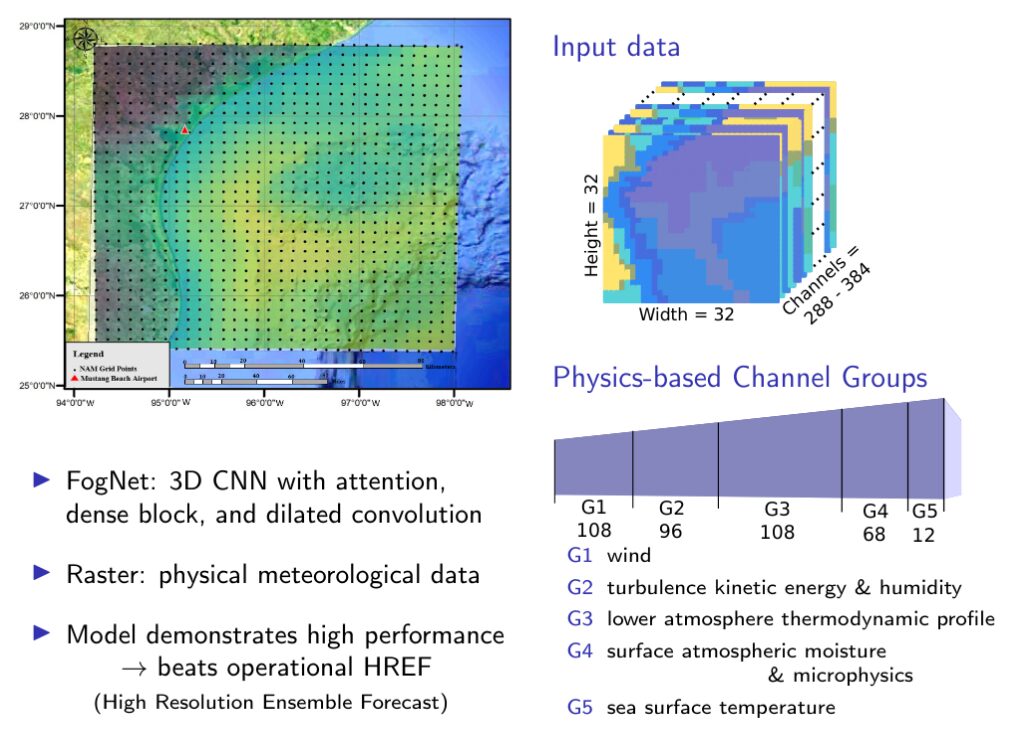
Highlighted Publications and Releases
We have yet to add these publications. In the mean time explore our publications.
AI Research to Operations: Semaphore
Semaphore is a python program that semi-automates the operationalizing AI models, at present models that can be saved as .H5 files. Semaphore uses an agile design, specific data objects, an easily expandable data ingestion module, and a database to support a wide variety of data series.
As of March 2024, Semaphore’s production server runs 55 operationalized models, including models related to coastal inundation and cold-stunning.
In January 2024, Semaphore supported the turtle cold-stunning NSF AI2ES operational model to guide port navigation, interrupt dredging, mobilize volunteers in the efforts to protect endangered turtle species in the Laguna Madre.
Semaphore is open-source and available on GitHub.
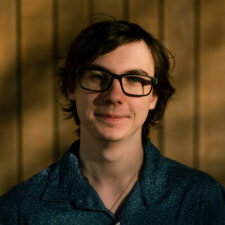
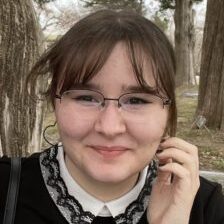
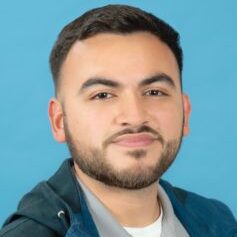
Highlighted Publications and Releases
Semaphore GitHub Repository Release
Physics-Inspired AI for Offshore Oceanography
This project focuses on developing advanced AI/ML models to enhance the prediction and understanding of ocean circulation and wave dynamics, specifically in the Gulf of Mexico and U.S. East Coast shelf seas. Our team is working to create a shared, high-resolution ocean circulation and wave dataset, design a deep learning platform to characterize air-sea-land interactions, and develop physics-based AI models to improve predictions of Loop Current Eddy shedding, Gulf Stream meanders, and ocean waves.
In the past few years, our team has developed OceanNet, a deep convolutional neural network with Fourier Neural Operators, and the OceanWave ML Ensemble, which outperforms conventional wave modeling approaches. OceanNet uses high-resolution ocean circulation reanalysis data from NCSU and provides superior forecasts compared to dynamical models for up to 120 days.
Related Project: Trustworthy AI for Ocean Eddy Prediction with Users
Highlighted Publications and Releases
A. Chattopadhyay, M. Gray, T. Wu, A. B. Lowe, and R. He. OceanNet: A principled neural operator-based digital twin for regional oceans. Scientific Reports, 14(1):21181, 2024. https://doi.org/10.1038/s41598-024-72145-0
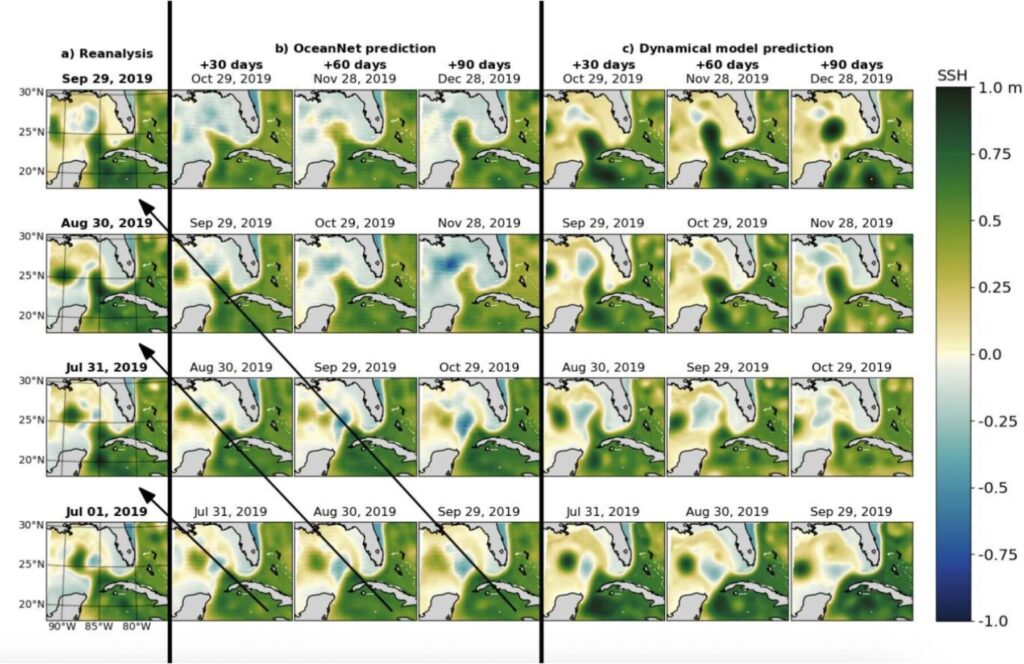
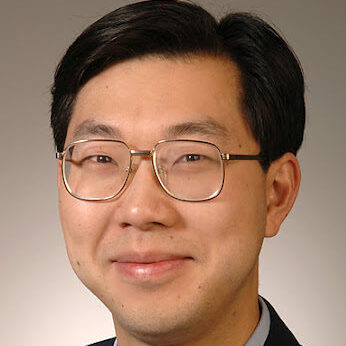
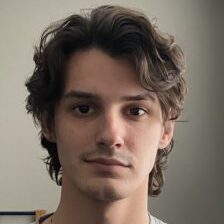
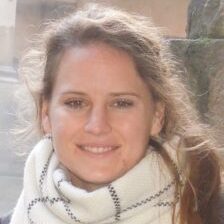
Advancing AI in Earth System Science Research
Join us to unlock AI’s potential in science.