Use-Inspired Research
Convective Weather
Advancing the tracking, prediction, and understanding of convective weather for improved forecasts and earlier emergency warnings.
Convective Initiation
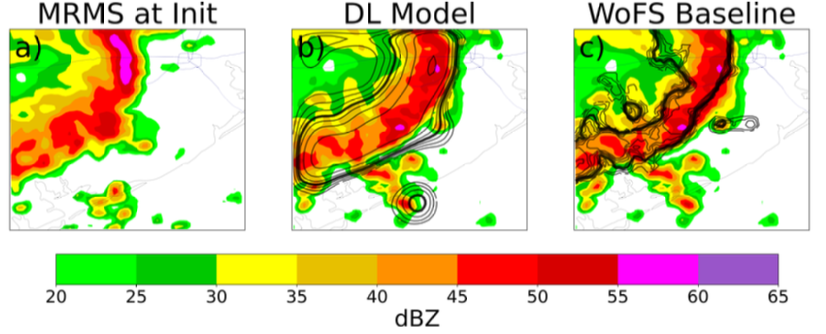
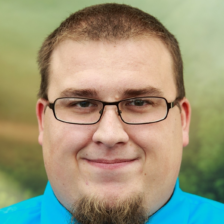
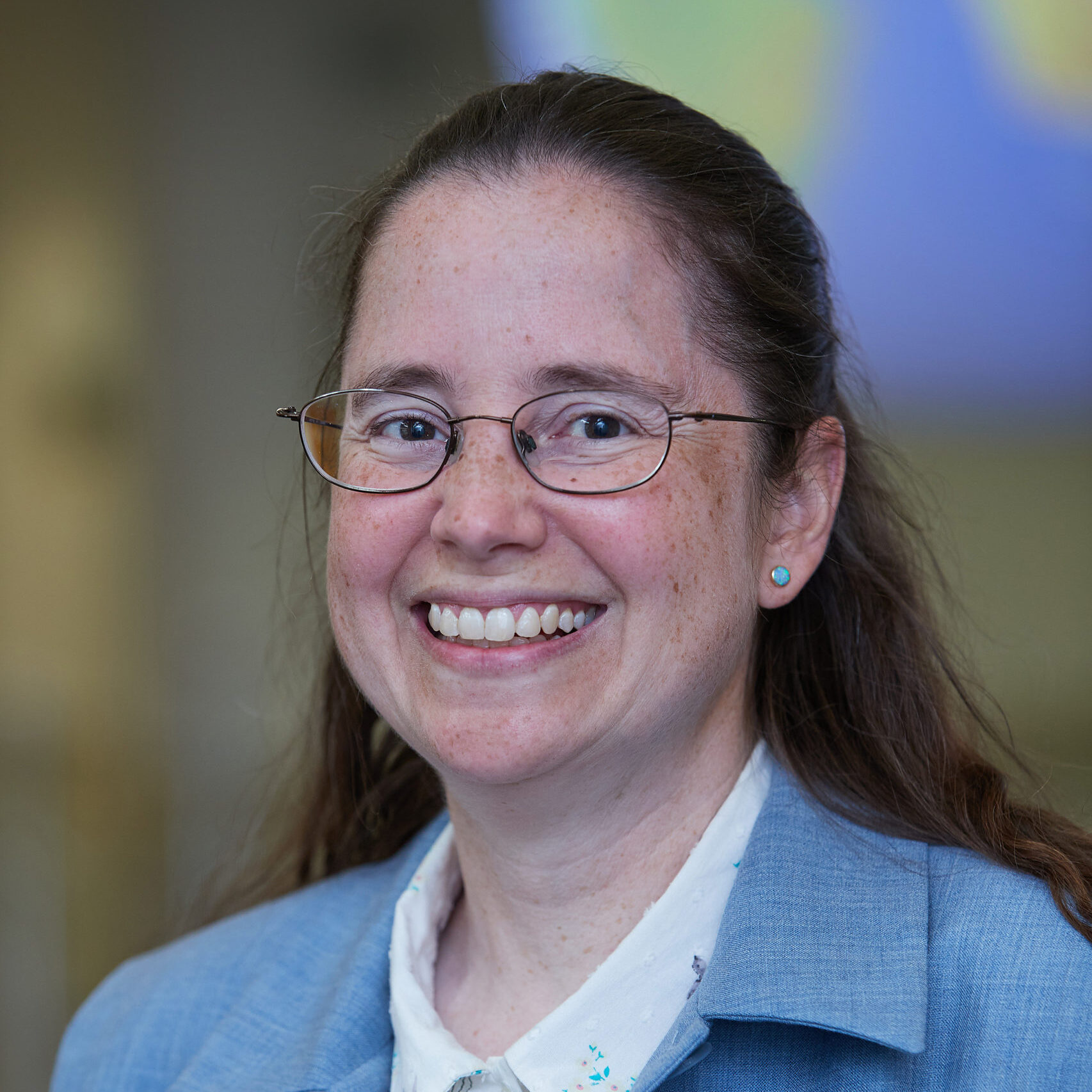
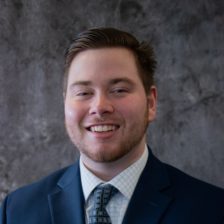
Our institute has been deeply embedded in improving the understanding and prediction of convective initiation and hazards while advancing XAI and deep learning methods for Earth system sciences. We developed a U-Net deep learning model to predict locations of thunderstorms trained on Warn-On-Forecast System (WoFS) model data consisting of environmental data. This model outperformed WoFS convection with a 30-minute lead time, providing insights into potential future improvements to WoFS. This work was done in collaboration with the National Severe Storms Laboratory (NSSL).
Furthermore, our team has developed a deep learning convective initiation model using satellite data and performed physically-informed XAI to analyze why it makes predictions with different levels of uncertainty. In addition we have produced through overviews on AI for convective weather at large, providing vital guidance to the great scientific community.
Convective weather is the prototype use-case for the synergistic cycle with AI & RC. We evaluated forecaster trust in two severe convective AI/ML products: a random forest model of hail guidance and a 3D-CNN model of storm model guidance. See the related project for more information.
Related Project: Trustworthy AI for Severe Convection with User Interviews
Highlighted Publications and Releases
Fan, D., S. J. Greybush, E. E. Clothiaux, and D. J. Gagne, 2024: Physically explainable deep learning for convective initiation nowcasting using GOES-16 satellite observations. Artificial Intelligence for the Earth Systems, In Press, https://doi.org/10.1175/AIES-D-23-0098.1.
A. M. Murphy, C. R. Homeyer, and K. Q. Allen. Development and investigation of GridRad-Severe, a multiyear severe event radar dataset. Monthly Weather Review, 151(9):2257–2277, Aug. 2023. doi:10.1175/MWR-D-23-0017.1.
A. McGovern, R. J. Chase, M. Flora, D. J. Gagne, R. Lagerquist, C. K. Potvin, N. Snook, and E. Loken. A review of machine learning for convective weather. Artificial Intelligence for the Earth Systems, 2:e220077, July 2023. doi:10.1175/AIES-D-22-0077.1.
Chad Wiley. Using Machine Learning to Improve the NSSL’s Warn-On-Forecast System’s Prediction of Thunderstorm Location. 2023, Master’s Thesis. ShareOK.
Chad Wiley (Presenter), Montgomery L. Flora, Corey K. Potvin, Amy McGovern. Improving the NSSL Warn-On-Forecast System (WoFS) Forecast of Convection Initiation Through Deep Learning. AMS Presentation. 2022.
Tornado Prediction in Real-time
Our researchers created novel studies of tornadoes from mesoscale convective systems, demonstrating key differences between the tornadoes that occur in MCSs versus super-cell tornadoes. Furthermore, we have developed a cloud-based, real-time tornado prediction model, tested in real-time using the WoFS ensemble alongside the 2023 NOAA’s Hazardous Weather Testbed’s (HWT) spring forecasting experiment. Currently, we are refining and evaluating our tornado prediction model, developing possible improvements via transfer learning.
Furthermore, our team developed real-time visualizations to enhance ML forecast interpretability, including plots intended to help forecasters assess ensemble forecast uncertainty and spatiotemporal variability (see figure).
Related Project: Trustworthy AI for Winter Precipitation Type with Users
Highlighted Publications and Releases
A. M. Murphy and C. R. Homeyer. Comparison of radar-observed tornadic and nontornadic MCS cells using probability-matched means. Journal of Applied Meteorology and Climatology, 62(10):1371–1388, Oct. 2023. doi:10.1175/JAMC-D-23-0070.1.
A. M. Murphy, C. R. Homeyer, and K. Q. Allen. Development and investigation of GridRad-Severe, a multiyear severe event radar dataset. Monthly Weather Review, 151(9):2257–2277, Aug. 2023. doi:10.1175/MWR-D-23-0017.1.
WoFS UNet Tornado Guidance Viewer (Website). NCAR MILES.
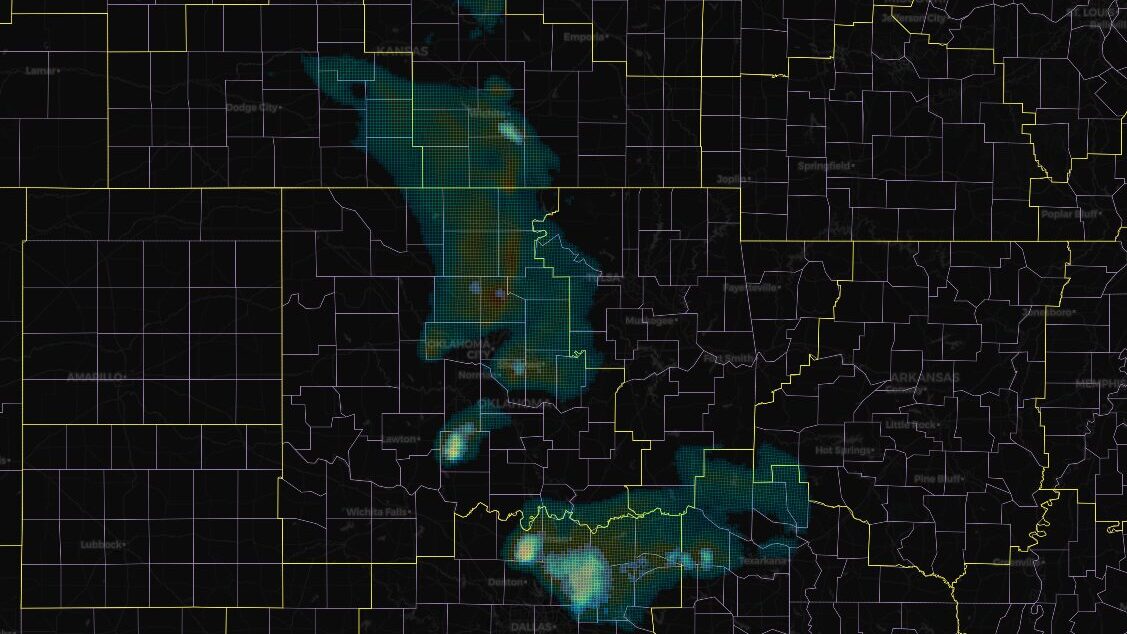
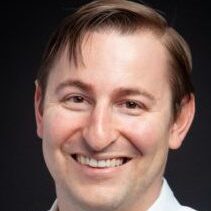
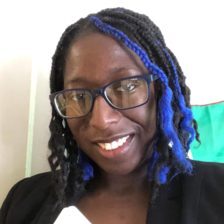
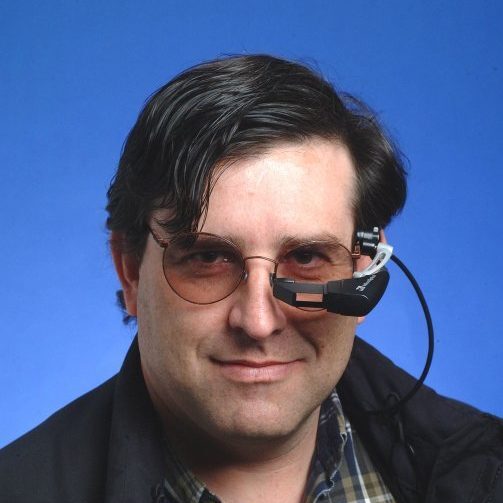
Hail Nowcasting
In a collaboration with government researchers at NOAA’s National Severe Storms Laboratory and private industry researchers at Vaisala, NSF AI2ES created an AI approach to nowcasting hail and convective initiation. The prototype hail prediction model developed here at the institute was further improved by an NSF AI2ES student through an internship at Vaisala. This work provided a better understanding the role of lightning during storm lifecycles and the likelihood of hail using the the hail prediction model. These models are currently being operationalized and refined into a product at Vaisala.
Related Project: Trustworthy AI for Severe Convection with User Interviews
Highlighted Publications and Releases
T. G. Schmidt, A. McGovern, J. T. Allen, C. K. Potvin, R. J. Chase, C. M. Wiley, W. R. McGovern-Fagg, M. L. Flora, C. R. Homeyer, and J. K. Williams. Gridded severe hail nowcasting using 3D U-Nets, lightning observations, and the Warn-on-Forecast system. Artificial Intelligence for the Earth
Systems, 2024. DOI:10.1175/AIES-D-24-0026.1
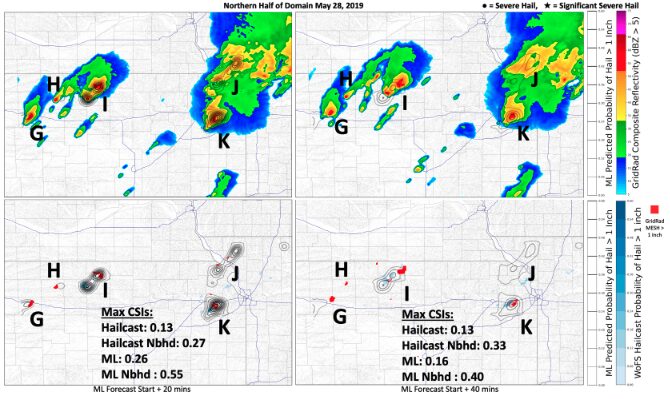
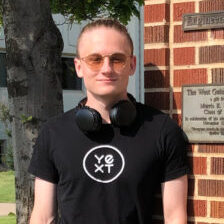
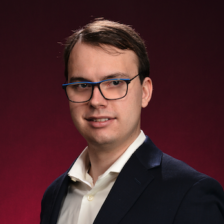
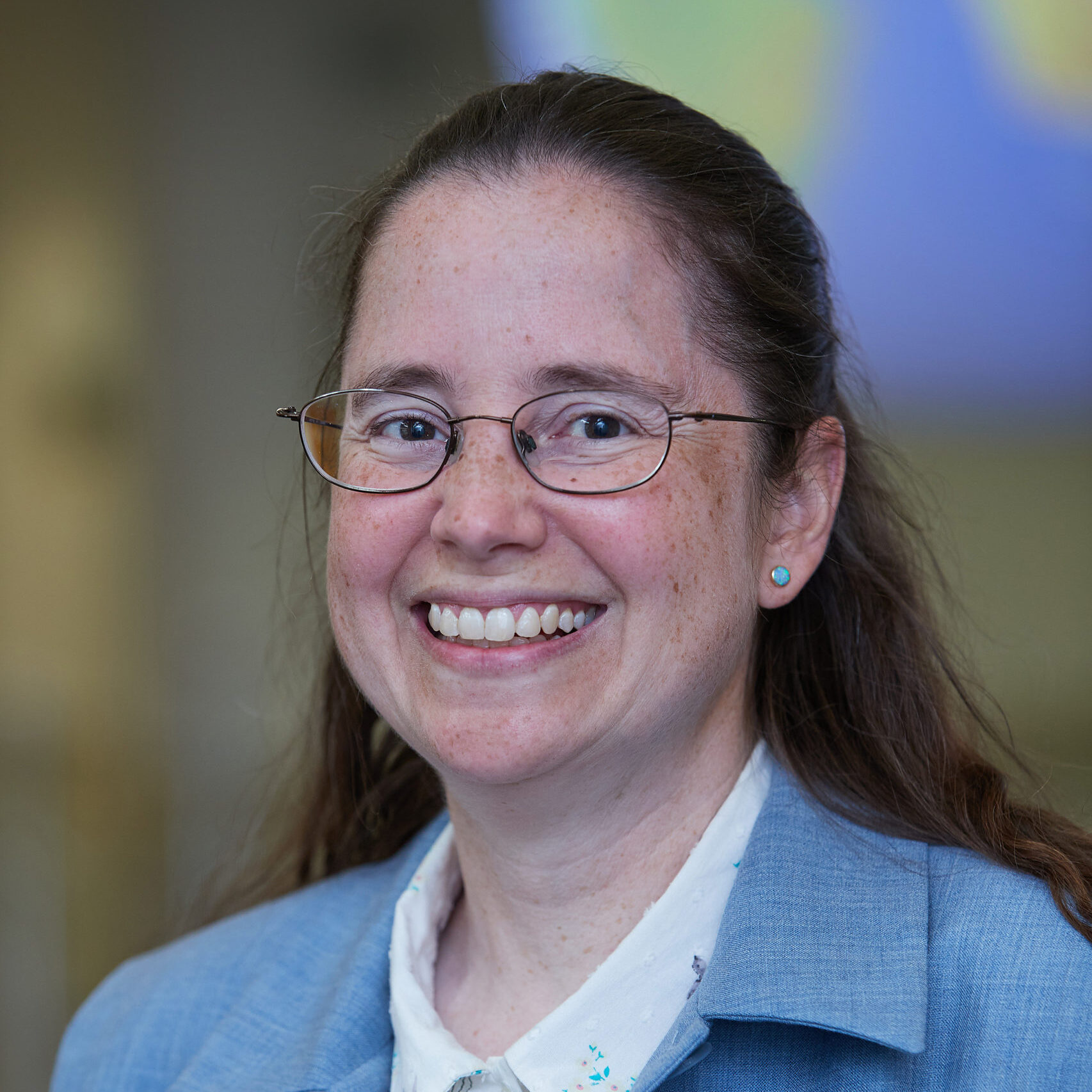
Advancing AI in Earth System Science Research
Join us to unlock AI’s potential in science.