Use-Inspired Research
Tropical Cyclones
Improving the prediction and understanding of tropical cyclone storm systems through AI/ML methods, advancing science discovery and forecast quality.
Synthetic Passive Microwave Imagery for Tropical Cyclone Science Discovery
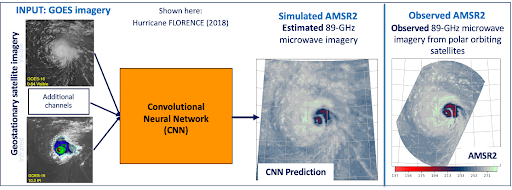
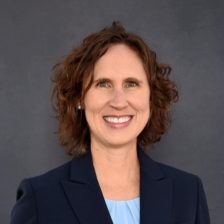
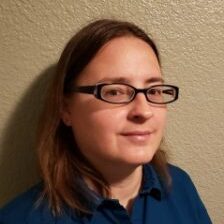
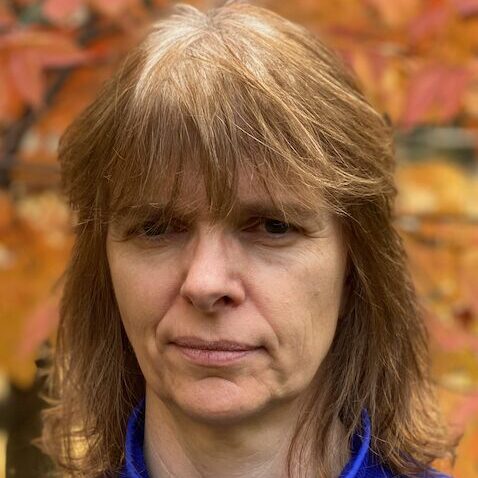
Geostationary satellites, unequipped with microwave instrumentation, cannot see through the high clouds in the atmosphere, obscuring vital information on the formation and evolution of tropical cyclones. However, passive microwave imagery can penetrate through the upper level clouds, providing valuable insights to tropical cyclone storm systems. Even so, hemispheric passes of these polar-orbiter microwave satellites are limited, providing only narrow swaths over the globe. Our team has developed a method of generating synthetic passive microwave (SPM) imagery of tropical cyclone systems from traditional geostationary satellite imagery, revealing the previously unseen internal structure of tropical cyclones at high temporal resolution. For example, the eye of a storm maybe obscured in the geostationary image. By extracting the corresponding microwave imagery through our approach, forecasters and emergency managers could identify the eye of the storm much sooner. This work would provide (1) gain deeper understanding of tropical cyclone evolution (see below) and (2) improve TC forecasting abilities.
Highlighted Publications and Releases
AMS AI presentation:
Marie McGraw, K. Haynes, K. D. Musgrave, I. Ebert-Uphoff, C. Slocum, and J. Knaff, Exploring Tropical Cyclone Structure and Evolution with AI-based Synthetic Passive Microwave Data, 104th AMS Annual Meeting, Baltimore, MD, Jan 2024.
AMS Tropical presentation:
McGraw, M.C., K. Haynes, K.D. Musgrave, C.J. Slocum, I. Ebert-Uphoff, and J.A. Knaff. Exploring Tropical Cyclone Structure and Evolution with AI-based Synthetic Passive Microwave Data. 36th AMS Conference on Hurricanes and Tropical Meteorology, Long Beach, CA, May 2024.
Understanding Tropical Cyclone Evolution using SPM Imagery
Tropical cyclone structure and evolution is governed by complex atmospheric dynamics, thermodynamics, and physics. In addition, tropical cyclones are relatively rare events, meaning that we have limited observational data of these phenomena. With the additional temporal coverage of tropical cyclones provided by the synthetic passive microwave imagery dataset (see above), we can also use machine learning to further study the physical processes that govern tropical cyclones, thus using machine learning for science discovery. Some of the processes we are analyzing include symmetric versus asymmetric processes, diurnal variability, and storm intensification.
Our research scientist Marie McGraw was a visiting scientist and is an ongoing collaborator at the Data-Driven Atmospheric and Water Dynamics Group at the Université de Lausanne (UNIL). In addition to her ongoing work on analyzing the SPM imagery, Marie is developing an approach for tropical cyclone intensity prediction. She was invited to present at the AI for Good Discovery seminar as part of her collaboration with Tom Beucler (UNIL) on AI and tropical meteorology.
Highlighted Publications and Releases
“Exploring Tropical Cyclone Structure and Evolution with AI-Based Synthetic Passive Microwave Data”. McGraw, M.C., K. Haynes, K.D. Musgrave, I. Ebert-Uphoff, C.J. Slocum, and J.A. Knaff. 24th AI Conference, AMS Annual Meeting, New Orleans, LA, USA. January 2025.
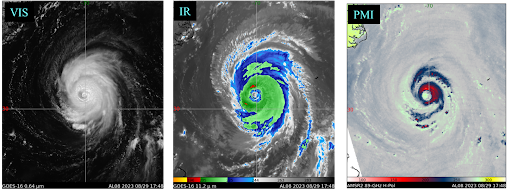
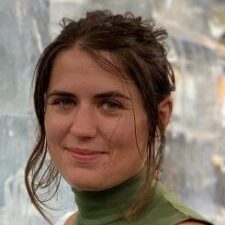
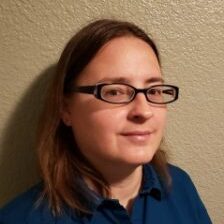
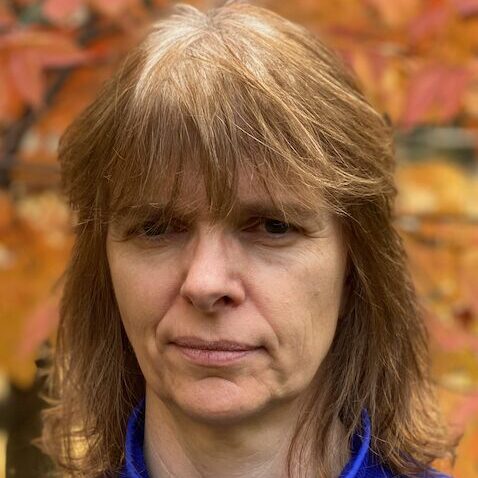
Uncertainty Estimates for Tropical Cyclones
Accurately estimating uncertainty in tropical cyclone forecasts is vital for effective disaster preparedness and response. Neural networks are increasingly used for these predictions, but providing reliable uncertainty estimates has been a challenge. Our team is currently working on novel ways to quantify and incorporate uncertainty into Earth system science applications, including tropical cyclone forecasting. We have devised a novel method using sinh-arcsinh-normal distributions to estimate heteroscedastic and asymmetric uncertainties, improving upon traditional techniques.
Select projects: Robust AI
Highlighted Publications and Releases
E. A. Barnes, R. J. Barnes, and M. DeMaria. Sinh-arcsinh-normal distributions to add uncertainty to neural network regression tasks: Applications to tropical cyclone intensity forecasts. Environmental Data Science, 2:e15, June 2023. doi:10.1017/eds.2023.7
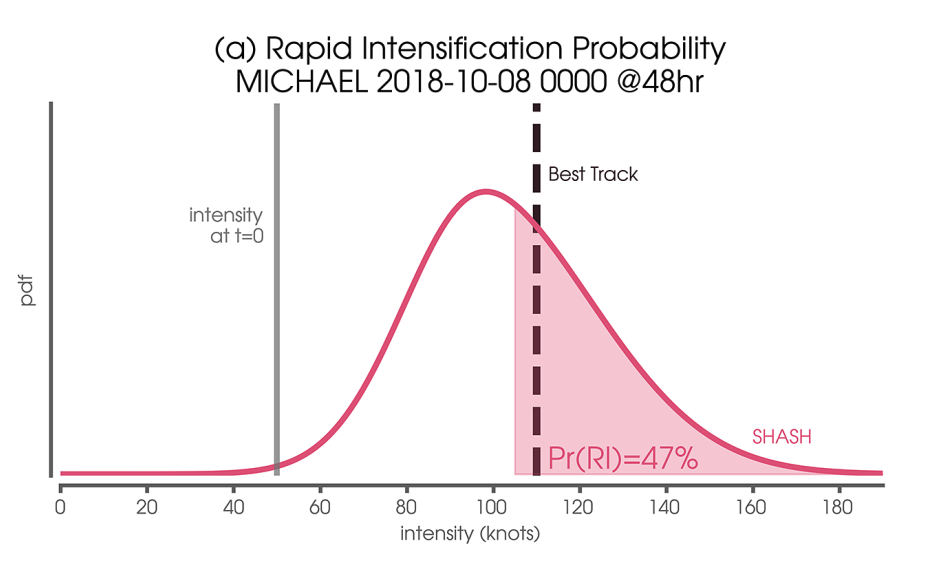
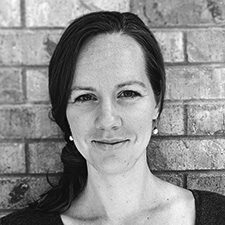
Advancing AI in Earth System Science Research
Join us to unlock AI’s potential in science.