Use-Inspired Research
S2S Prediction of Extreme Weather
Bridging the gap between short-term and long-term forecasts.
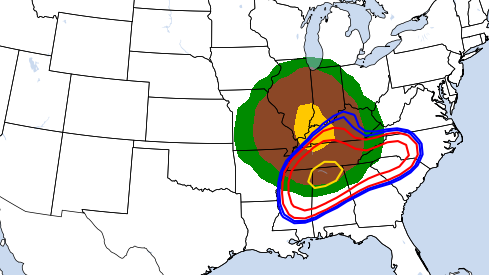
AI for S2S Scientific Discovery
Improving our understanding and expanding the predictability of convective severe weather across different regions of the United States.
Interpretable AI for S2S
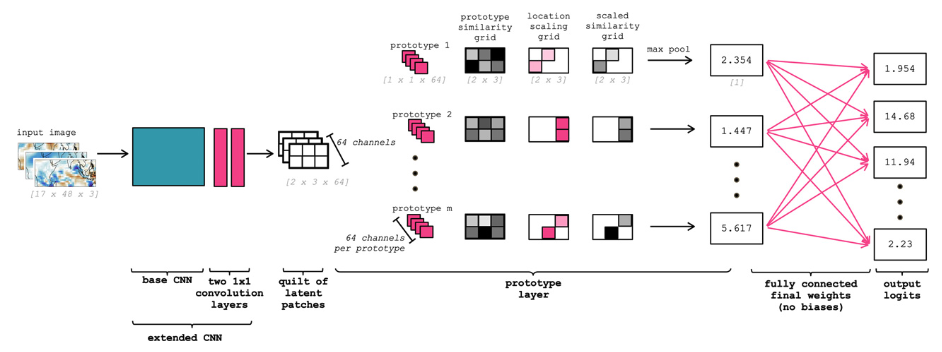
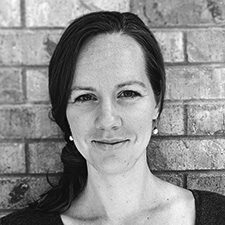
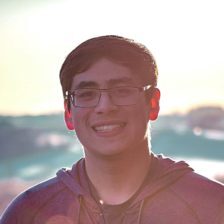
Our team has developed an interpretable AI approach for transfer learning to improve S2S prediction of temperature along the U.S. West Coast. The approach shows skill at S2S timescales (a timescale which is notoriously difficult to predict) where extensive observations are lacking for training on observations alone. We have also devised an interpretable AI approach to make skillful climate forecasts on subseasonal-to-decadal timescales via analog forecasting. We are exploring a different interpretable model approach for S2S prediction based on the method of “analogs” for NOAA.
We successfully deployed robust, interpretable and XAI methods to identify skillful “forecasts of opportunity” (i.e. states of the climate system that are more predictable than others). We demonstrated that by training on climate model output (CESM2) and then fine-tuning with as well as reanalysis/observations (ERA5) we can make skillful S2S forecasts of North American temperatures in the reanalysis. This approach led us to identify representative patterns (i.e. prototypical patterns) within the real-world that lead to strong tropical Madden-Julian oscillation teleconnections to the midlatitudes.
Our prototype-based interpretable neural network when location matters (i.e. that is applicable to geophysical applications) for use for S2S prediction can be found to the left (see figure).
Related Project: Explainable AI, Robust AI
Highlighted Publications and Releases
Gordillo, Nicolas J., and Elizabeth A. Barnes. 2024. “Application of an Interpretable Prototypical-Part Network to Subseasonal-to-Seasonal Climate Prediction over North America”, under review.
Rader, Jamin K., and Elizabeth A. Barnes. 2023. “Optimizing Seasonal-To-Decadal Analog Forecasts With a Learned Spatially-Weighted Mask.” Geophysical Research Letters 50 (23): e2023GL104983. https://doi.org/10.1029/2023GL104983.
Barnes, Elizabeth A., Randal J. Barnes, Zane K. Martin, and Jamin K. Rader: This Looks Like That There: Interpretable neural networks for image tasks when location matters, Artificial Intelligence for the Earth Systems, 2022.
Barnes, Elizabeth A. and Randal J. Barnes: Controlled abstention neural networks for identifying skillful predictions for regression problems, Journal of Advances in Modeling Earth Systems, https://doi.org/10.1029/2021MS002575.
Barnes, Elizabeth A. and Randal J. Barnes: Controlled abstention neural networks for identifying skillful predictions for classification problems, Journal of Advances in Modeling Earth Systems, https://doi.org/10.1029/2021MS002573.
AI for S2S Scientific Discovery
We use deep learning within a scientific discovery framework to improve our understanding and expand the predictability of convective severe weather across different regions of the United States. We use a convolutional neural network to assess the likelihood of severe weather hazards, including tornadoes, hail, and wind, over timescales ranging from one to three weeks. Then, using explainable artificial intelligence frameworks, we can identify key features and locations within the input data that significantly influence the model predictions. The integration of deep learning techniques in a scientific discovery framework provides valuable insights into the dynamical processes and teleconnections that contribute to enhanced predictability of severe convective weather.
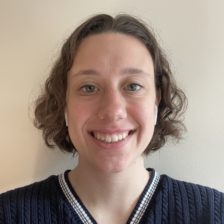
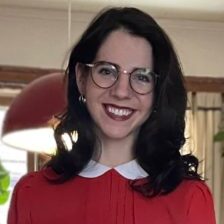
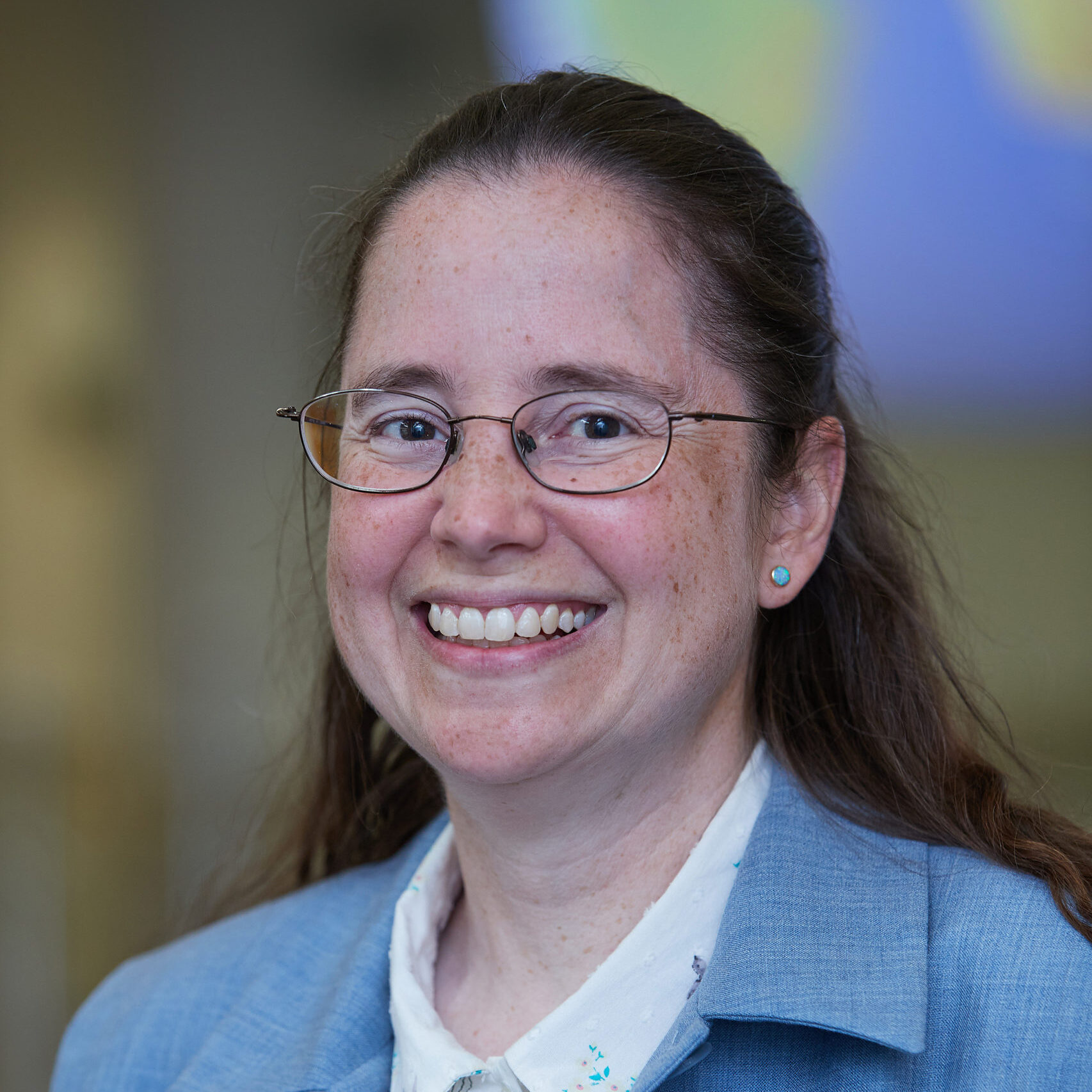
Highlighted Publications and Releases
E. Salm, M. Madsen, A. McGovern. Using Machine Learning Methods to Predict and Understand Severe Weather Over the United States. AMS Conference 2025. https://ams.confex.com/ams/105ANNUAL/meetingapp.cgi/Paper/455409
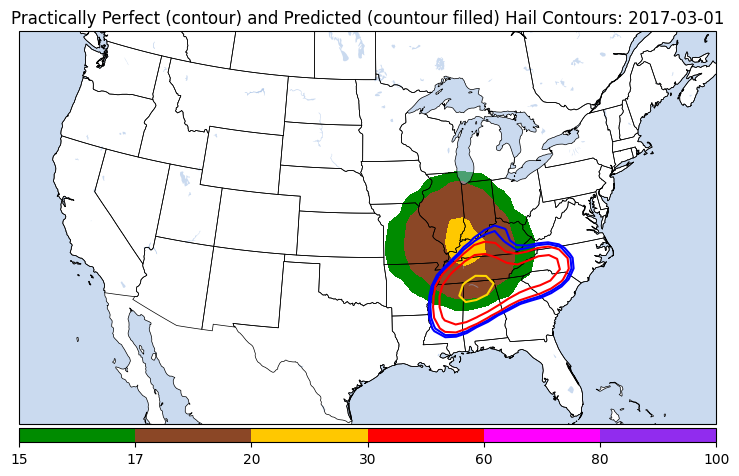
Water Level Prediction
We a new method to predict average regional water levels along the Northwest Gulf of Mexico for S2S to multi-year predictions. While deep Gulf of Mexico heat profiles were expected to be skillful predictors, the method is presently only based on past processed regional average water levels, the former likely still driving the system at the S2S scale. This work was done in collaboration with the University of Valencia, Spain.
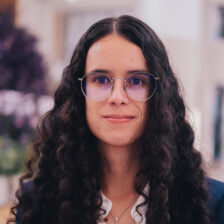
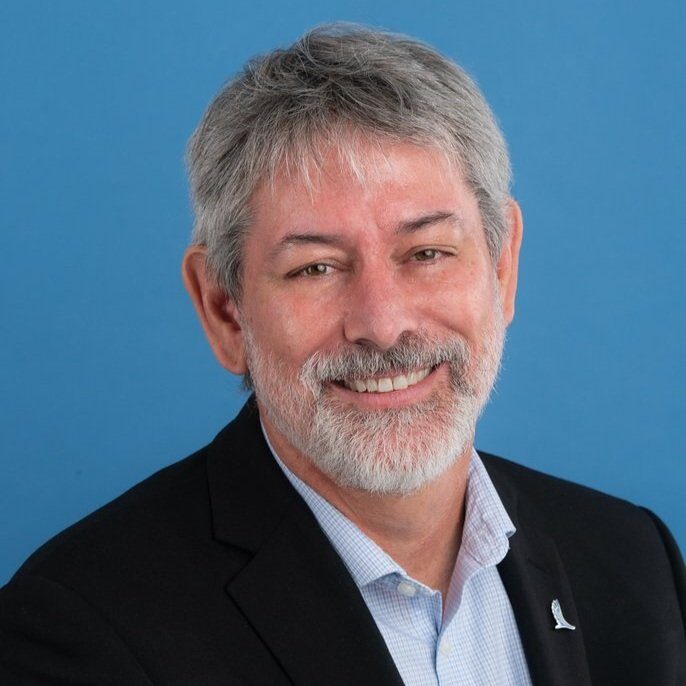
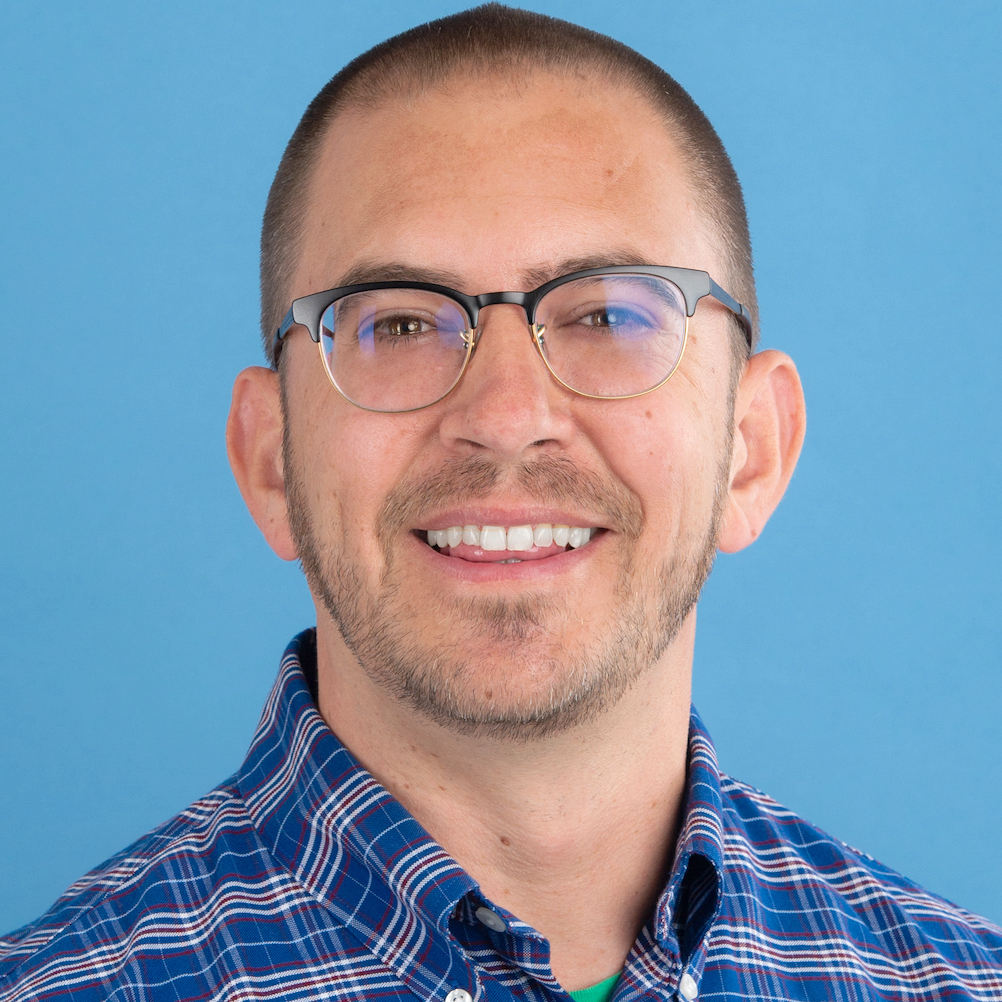
Highlighted Publications and Releases
Vicens-Miquel, M.; Radin, C.; Nieves, V.; Tissot, P.. Towards Resilient Coastal Management: Supervised Learning for Seasonal to Multi-Year Water Level Predictions in Texas Gulf Coast. Frontiers in Earth Science, section Geoinformatics, In submission.
Vicens-Miquel, M.; Radin, C.; Nieves, V.; Tissot, P.; Medrano, A. Advancing Coastal Inundation Frequency Predictions with an AI-based Sub-seasonal to Multi-year Water Level Model in the Gulf of Mexico. Presented at the AGU Fall Meeting 2023, San Francisco, CA, USA, December 11-15.
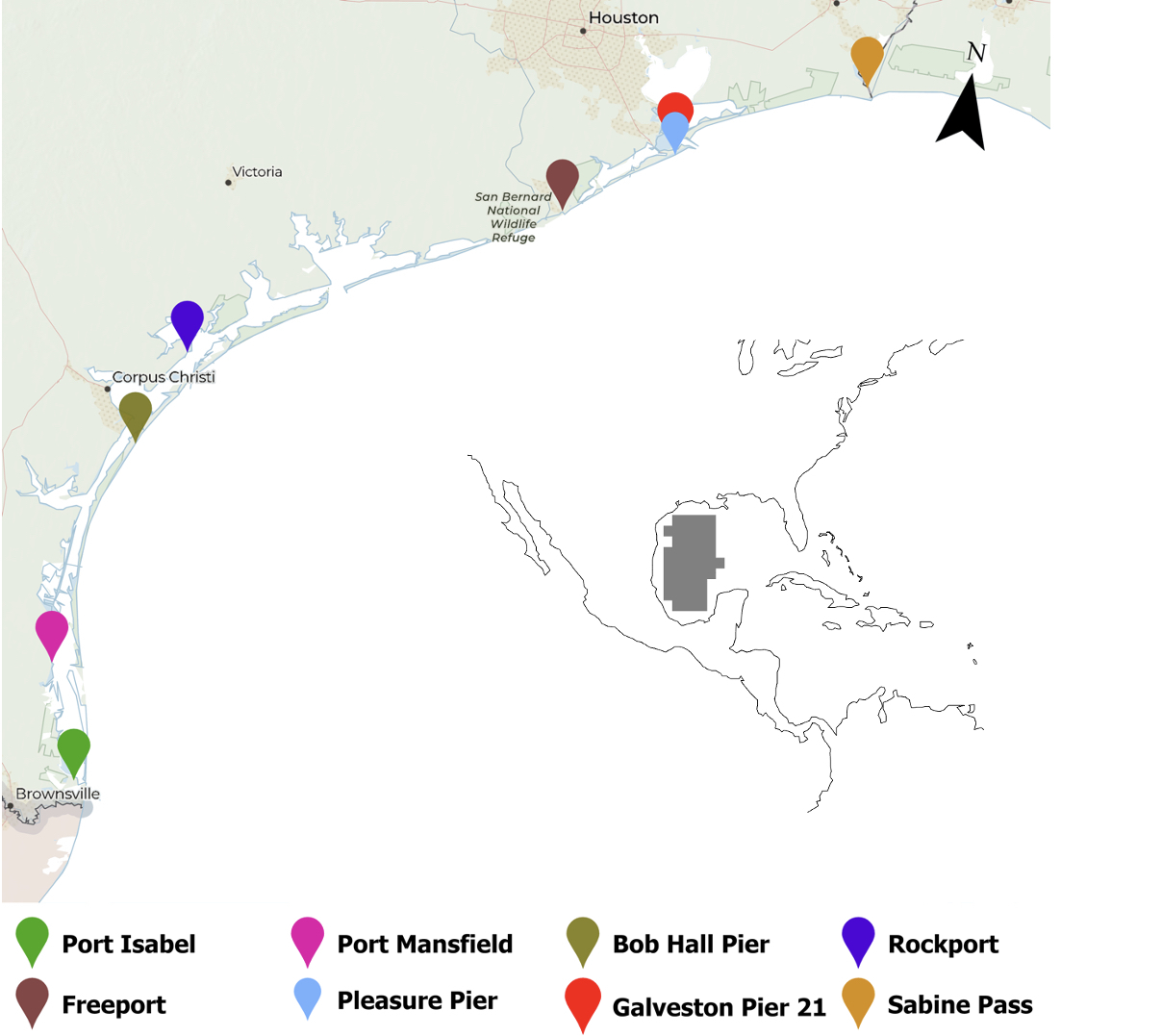
Advancing AI in Earth System Science Research
Join us to unlock AI’s potential in science.