Use-Inspired Research
Winter Weather
Advancing our understanding of winter weather patterns and enhancing their predictability across synoptic to local scales, providing local guidance to improve community safety and state-wide transportation.
Winter Road Weather: Decision Making
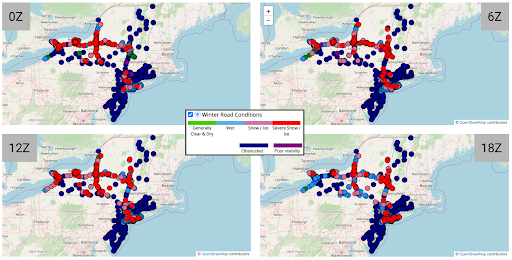
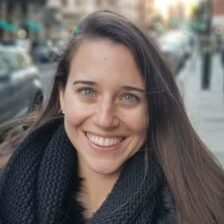
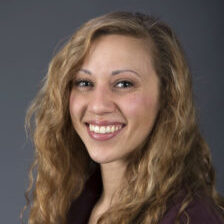
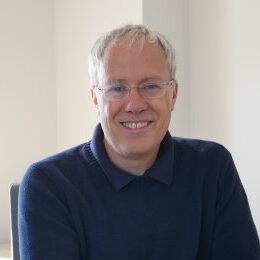
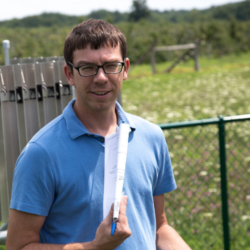
Severe winter weather poses significant challenges for roadway safety, increasing the risk of accidents and disrupting transportation systems. To address this, our winter weather team has developed an AI model that provides real-time, automated identification and prediction of road weather conditions for the New York Department of Transportation (NYSDOT). This model leverages a comprehensive dataset created in collaboration with NYSDOT, which includes archived images from 2,400 live 511NY traffic cameras. Our solution will deliver real-time decision-support tools to help NYSDOT rapidly assess and respond to hazardous winter road conditions. By enhancing situational awareness and enabling faster, data-driven decisions, this project aims to improve public safety, reduce weather-related delays, and optimize road maintenance operations across New York State.
Related Project: Leveraging Social Science for Reproducible Supervised ML Hand Labeling
Highlighted Publications and Releases
[In preparation] Sutter, C., Sulia, K., Bassill, N. P., Wirz, C. D., Przybylo, V., Cains, M. G., Radford, J., Evans, D. A, Rothenberger, J., Thorncroft, C. D. (2024) Automated Classification of Road Surface Conditions using New York State Department of Transportation Camera Images and Weather Forecast Data with Machine Learning Methods.
Wirz, C. D., Sutter, C., Demuth, J. L., Mayer, K. J., Chapman, W. E., Cains, M. G., et al. (2024). Increasing the reproducibility and replicability of supervised AI/ML in the Earth systems science by leveraging social science methods. Earth and Space Science, 11, e2023EA003364. https://doi.org/10.1029/2023EA003364)
Sutter, C., Sulia, K.J., Bassill, N.P. , Thorncroft, C.D., Przybylo, V., Wirz, C.D., Cains, M.G., Radford, J.T., Evans, D.A. (2024) Improving Generalizability of Road Condition Classification Models for Department of Transportation Camera Images, 104th AMS Annual Meeting, Baltimore, MD, Jan 2024. (https://ams.confex.com/ams/104ANNUAL/meetingapp.cgi/Paper/438154) [presented by Dr. Kara Sulia]
Sutter, C., Sulia, K.J, Bassill, N. P., Thorncroft, C. D., Wirz, C. D., Przybylo, V., Cains, M. G., Radford, J., & Evans, D. A. (2023). Quantitative Content Analysis Data for Hand Labeling Road Surface Conditions in New York State Department of Transportation Camera Images (1.0.0) [Data set]. Zenodo. (https://doi.org/10.5281/zenodo.8370665)
Bostrom, A., Demuth, J., Wirz, C., Cains, M., Schumacher, A., Madlambayan, D., Bansal, A. S., Bearth, A., Chase, R., Crosman, K.M., Ebert-Uphoff, I., Gagne II, D.J., Guikema, S., Hoffman, R., Johnson, B.B., Kumler-Bonfanti, C., Lee, J.D., Lowe, A., McGovern, A., Przybylo, V., Radford, J., Roth, E., Sutter, C., Tissot, P., Roebber, P., Stewart, J.Q., White, M., & Williams, J.K. (2023). Trust and trustworthy artificial intelligence: A research agenda for AI in the environmental sciences. Risk Analysis, online first, (https://doi.org/10.1111/risa.14245)
Sutter, C., Sulia, K.J., Przybylo, V, Bassill, N.P., Thorncroft, C.D., Wirz, C.D., Cains, M.G., (2023) Automated Detection of Road Conditions from Department of Transportation Camera Images [Conference presentation]. American Meteorological Society 103rd Annual Meeting, Denver, CO, 8-12 January 2023 (https://ams.confex.com/ams/103ANNUAL/meetingapp.cgi/Paper/418646 )
V.A. Ferrera, J.C. Rothenberger, M.W. Reyes, C. Sutter, A.H. Fagg, and D.I. Diochnos. Classifying road surface conditions with self-trained artificial intelligence. In 103rd AMS Annual Meeting. AMS, 2023.
J. C. Rothenberger, T. Le, C. Sutter, K. J. Sulia, and D. Diochnos. Improving road surface classification with co-training algorithms. In 105rd AMS Annual Meeting. AMS, 2025.
Precipitation
Winter Weather P-Type Analysis and Forecasts
Accurate winter precipitation-type (p-type) forecasts are critical for public safety and effective decision-making during hazardous weather events. This project aims to improve the accuracy, quantify uncertainty, and ensure the physical consistency of winter weather p-type analyses and forecasts. We are developing an advanced AI-based p-type system, along with tailored visualizations and documentation, to deliver actionable information for decision-makers across various forecast lead times.
Key achievements include the development of an evidential p-type model that provides both high accuracy and robust uncertainty estimates. We have successfully applied this model to High-Resolution Rapid Refresh (HRRR) hindcasts. Additionally, we are refining an interview protocol in collaboration with our Risk Communication team and building a visualization website to enhance communication of p-type hindcasts. The project also supports workforce development through mentorship of multiple interns, including three SIParCS interns (two in 2023, one in 2024) and a Vaisala intern in 2023.
Related Project: Trustworthy AI for Winter Precipitation Type with Users
Highlighted Publications and Releases
J. S. Schreck, D. J. Gagne, C. Becker, W. E. Chapman, K. Elmore, D. Fan, G. Gantos, E. Kim, D. Kimpara, T. Martin, M. J. Molina, V. M. Przybylo, J. Radford, B. Saavedra, J. Willson, and C. Wirz. Evidential deep learning: Enhancing predictive uncertainty estimation for Earth system science applications. Artificial Intelligence for the Earth Systems, 2024. doi:10.1175/AIES-D-23-0093.1.
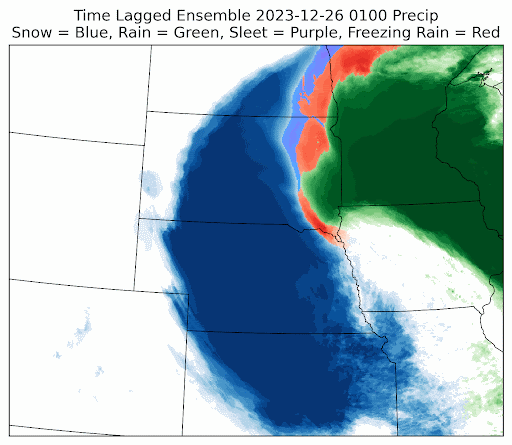
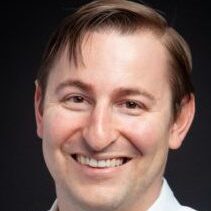
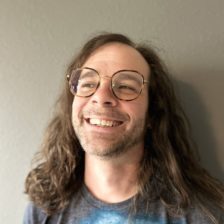
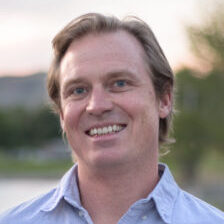
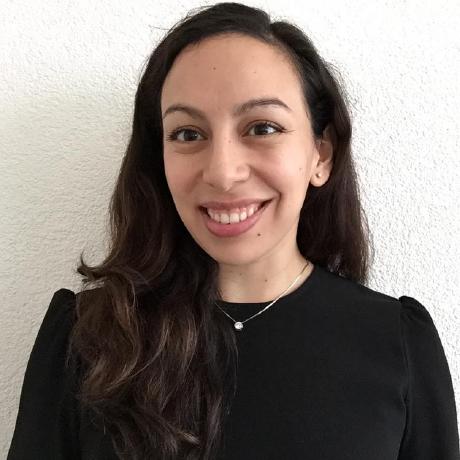
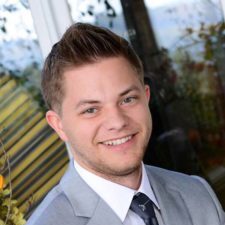
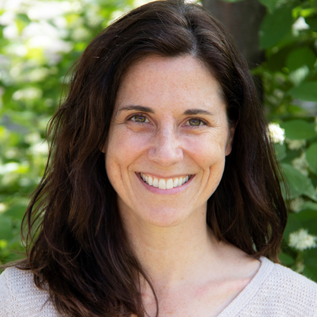
Precipitation Detection from Cameras
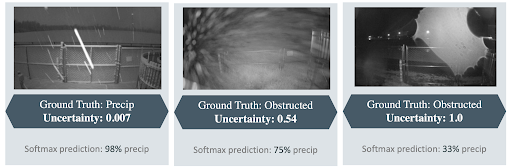
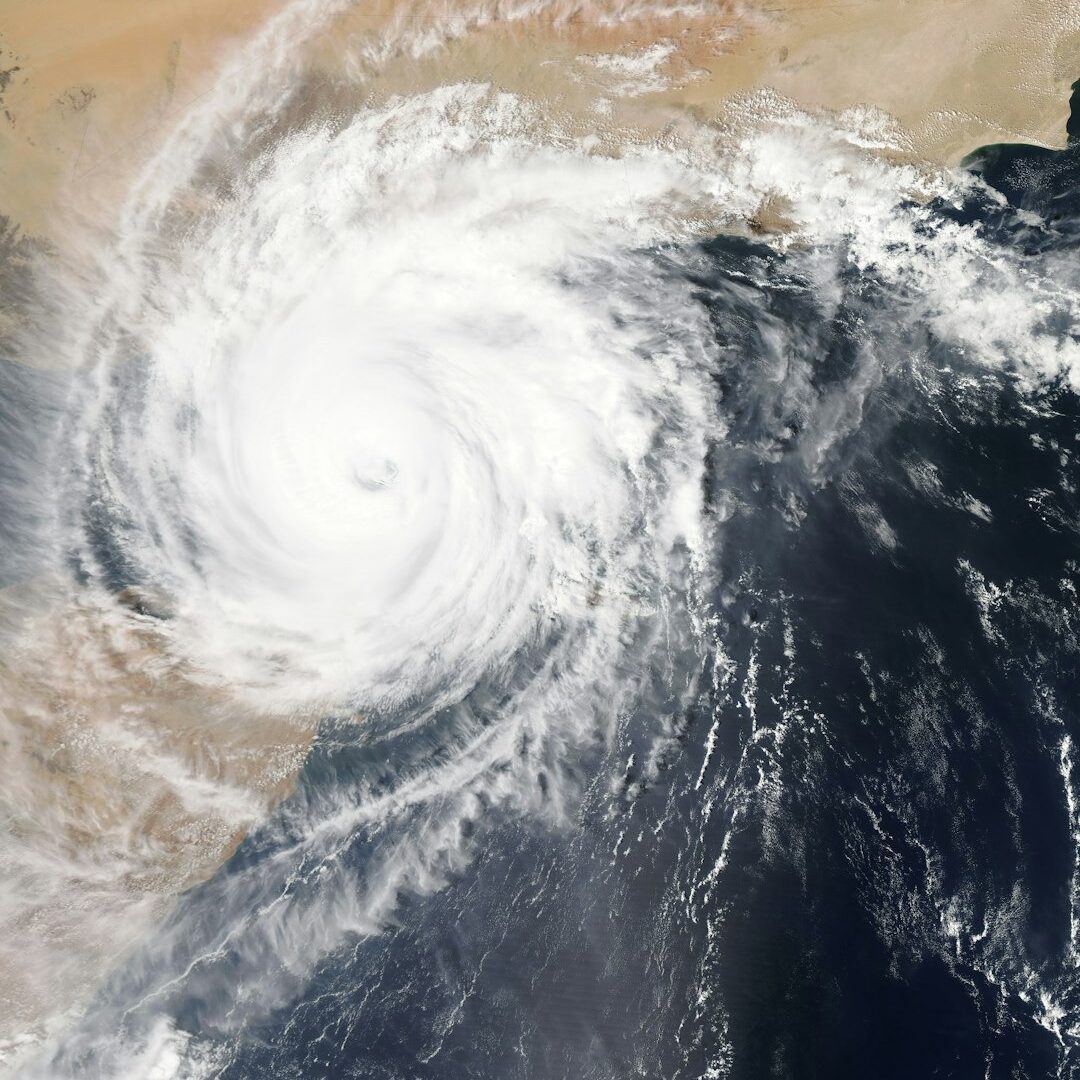
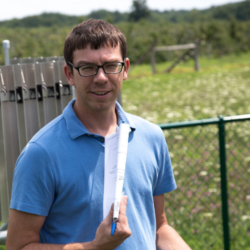
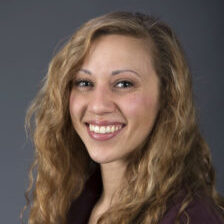
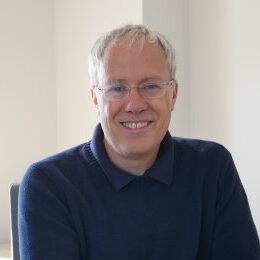
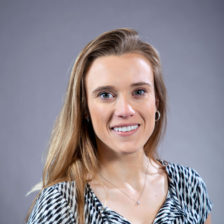
Accurately detecting precipitation in real-time can assist in state-wide transportation and operations, especially during cold and icy winter conditions. This project aims to develop an AI-powered system that uses explainable and trustworthy methods to detect the presence or absence of precipitation streaks in New York State Mesonet (NYSM) camera images during both daytime and nighttime. Integrated with the NYSM web-based interface, this automated detection system will provide real-time insights for weather monitoring. Our Winter Weather team is collaborating with the Albany Weather Forecast Office (WFO) to align with operational needs, and establishing robust labeling methods for training machine learning models.
Related Project: Leveraging Social Science for Reproducible Supervised ML Hand Labeling
Highlighted Publications and Releases
Horan, B.T., Sulia, K.J., Bassill, N.P. , Thorncroft, C.D., Sutter, C., Evans, D.A. (2025) Creating Convolutional Neural Networks to Monitor New York State Mesonet Daytime Precipitation from Camera Images, 105th AMS Annual Meeting, New Orleans, LA, Jan 2025. (https://ams.confex.com/ams/105ANNUAL/meetingapp.cgi/Paper/450195)
Classification of NYSM Imagery GitHub repository: https://github.com/vprzybylo/ai2es?trk=public_profile_project-button
J. S. Schreck, D. J. Gagne, C. Becker, W. E. Chapman, K. Elmore, D. Fan, G. Gantos, E. Kim, D. Kimpara, T. Martin, M. J. Molina, V. M. Przybylo, J. Radford, B. Saavedra, J. Willson, and C. Wirz. Evidential deep learning: Enhancing predictive uncertainty estimation for Earth system science applications. Artificial Intelligence for the Earth Systems, 2024. doi:10.1175/AIES-D-23-0093.1.
Bostrom, A., Demuth, J., Wirz, C., Cains, M., Schumacher, A., Madlambayan, D., Bansal, A. S., Bearth, A., Chase, R., Crosman, K.M., Ebert-Uphoff, I., Gagne II, D.J., Guikema, S., Hoffman, R., Johnson, B.B., Kumler-Bonfanti, C., Lee, J.D., Lowe, A., McGovern, A., Przybylo, V., Radford, J., Roth, E., Sutter, C., Tissot, P., Roebber, P., Stewart, J.Q., White, M., & Williams, J.K. (2023). Trust and trustworthy artificial intelligence: A research agenda for AI in the environmental sciences. Risk Analysis, online first, (https://doi.org/10.1111/risa.14245)
Visibility Estimation from Camera Images
Accurate atmospheric visibility estimation is essential for ensuring public safety and improving decision-making during dangerous winter weather conditions. This project focuses on developing AI models to estimate visibility from a variety of camera sources, including the New York State Mesonet (NYSM) and Department of Transportation (DOT) networks. Our approach leverages machine learning techniques capable of handling labels of varying quality while incorporating human-in-the-loop refinement to enhance the accuracy and trustworthiness of visibility estimates. Key achievements include developing a convolutional neural network (CNN) that can compare relative visibility between image pairs and generalize to previously unseen cameras. For each NYSM station, we have curated a set of human-verified reference images with known visibility distances, enabling more precise model estimates across New York State. Using these trained models and reference images, we are developing a real-time visibility assessments through a visual interface that allows end users to monitor visibility conditions.
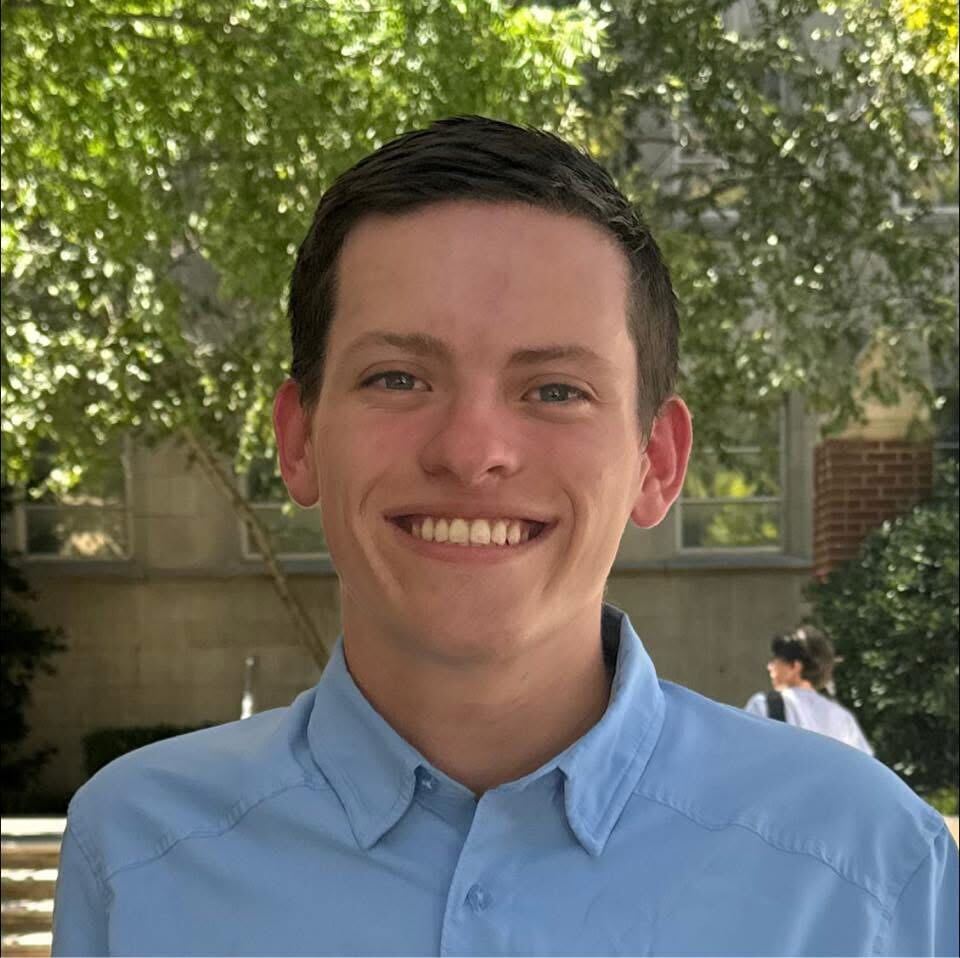
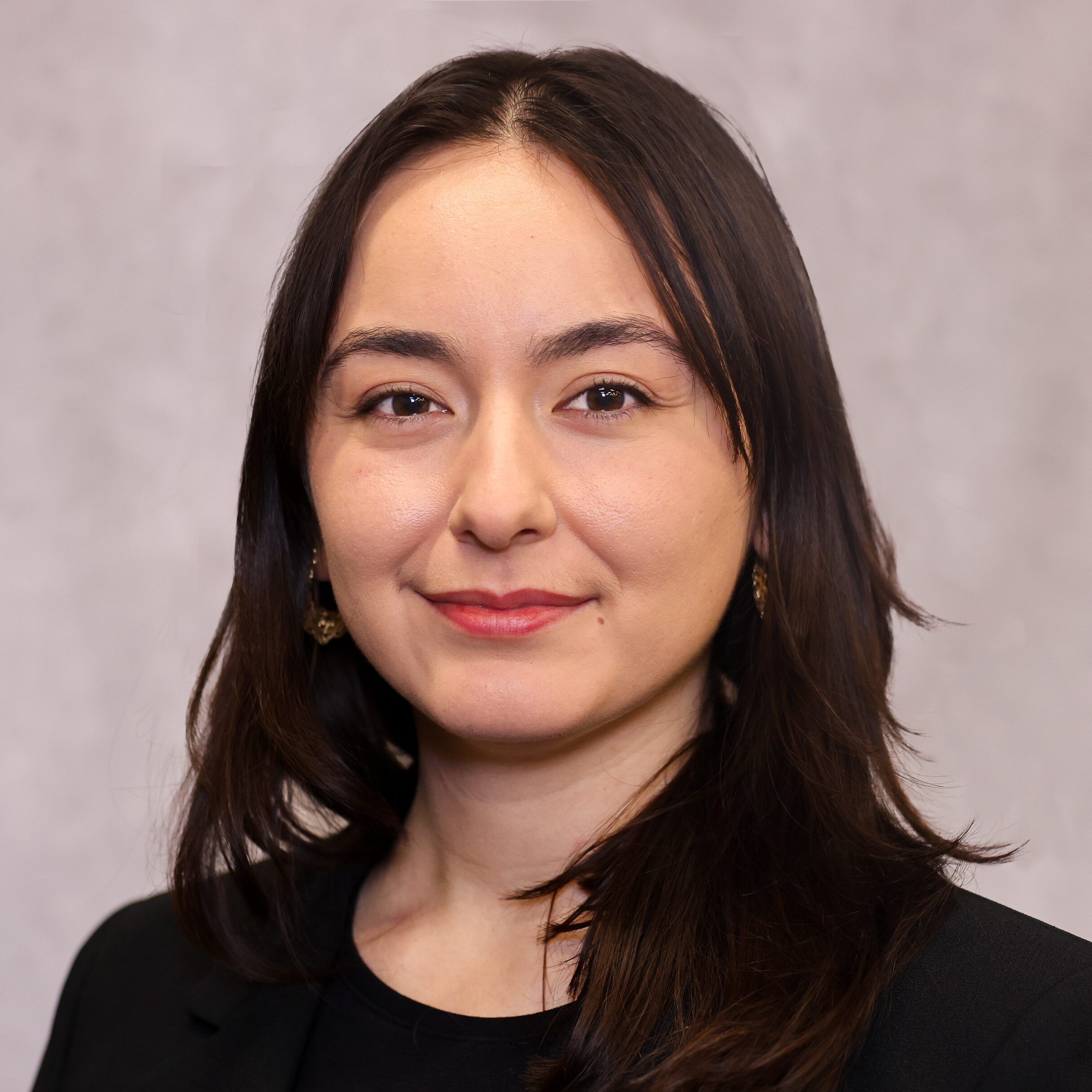
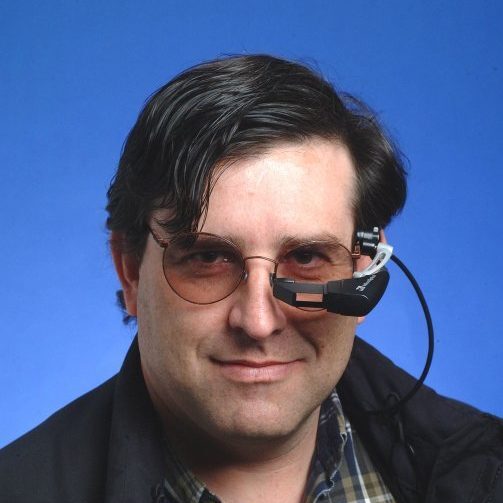
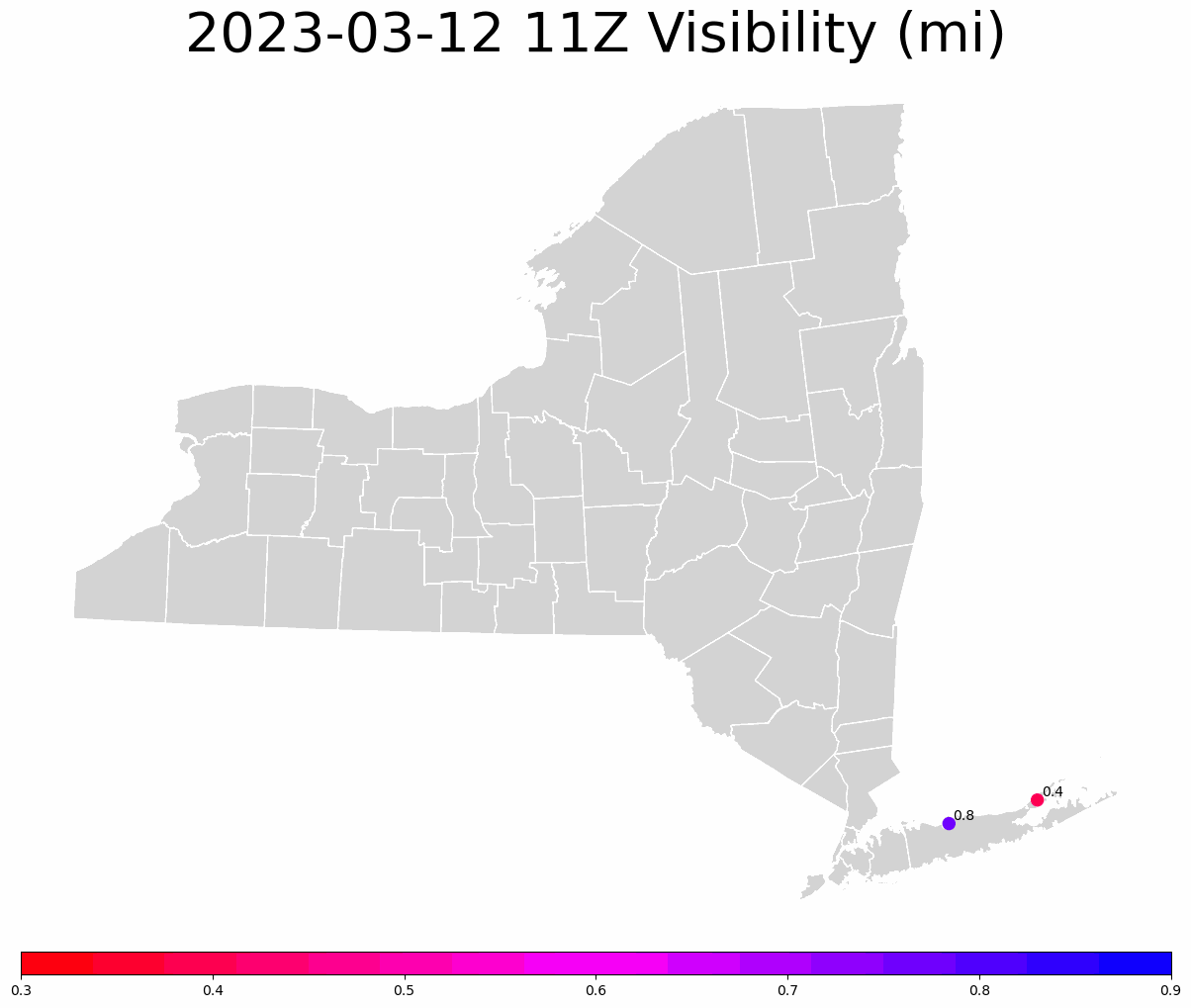
Highlighted Publications and Releases
M. Wilson Reyes, “Visibility Estimation from Camera Images Using Deep Learning,” Master’s Thesis. 2023. https://shareok.org/items/bfebee6b-5002-40d7-b93d-4d6892e5292d
M. Wilson Reyes, A. Kurbanovas, A.H. Fagg, C.D. Thorncroft, K.J. Sulia, J.A. Brotzge, “Generalized Visibility Estimation from Camera Images Using Deep Learning,” AMS 2024 (Conference Presentation)
M. Wilson Reyes, A. Kurbanovas, A.H. Fagg, C.D. Thorncroft, K.J. Sulia, J.A. Brotzge, “Comparative Visibility Estimation from New York State Mesonet Camera Images Using Deep Learning,” AMS 2023 (Conference Presentation)
Numerical Weather Prediction Uncertainty Quantification
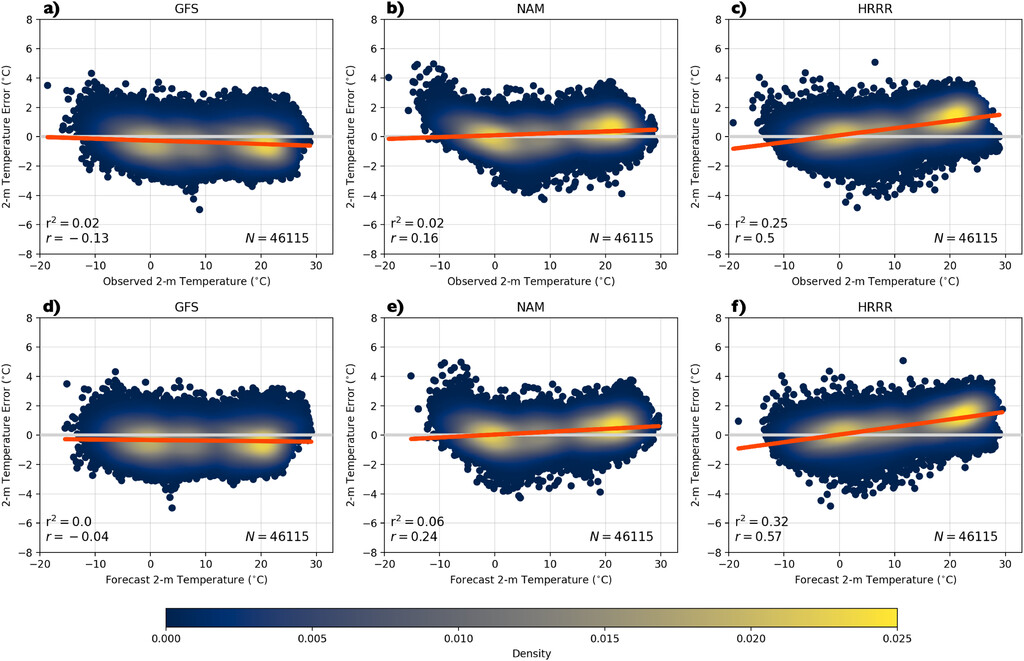
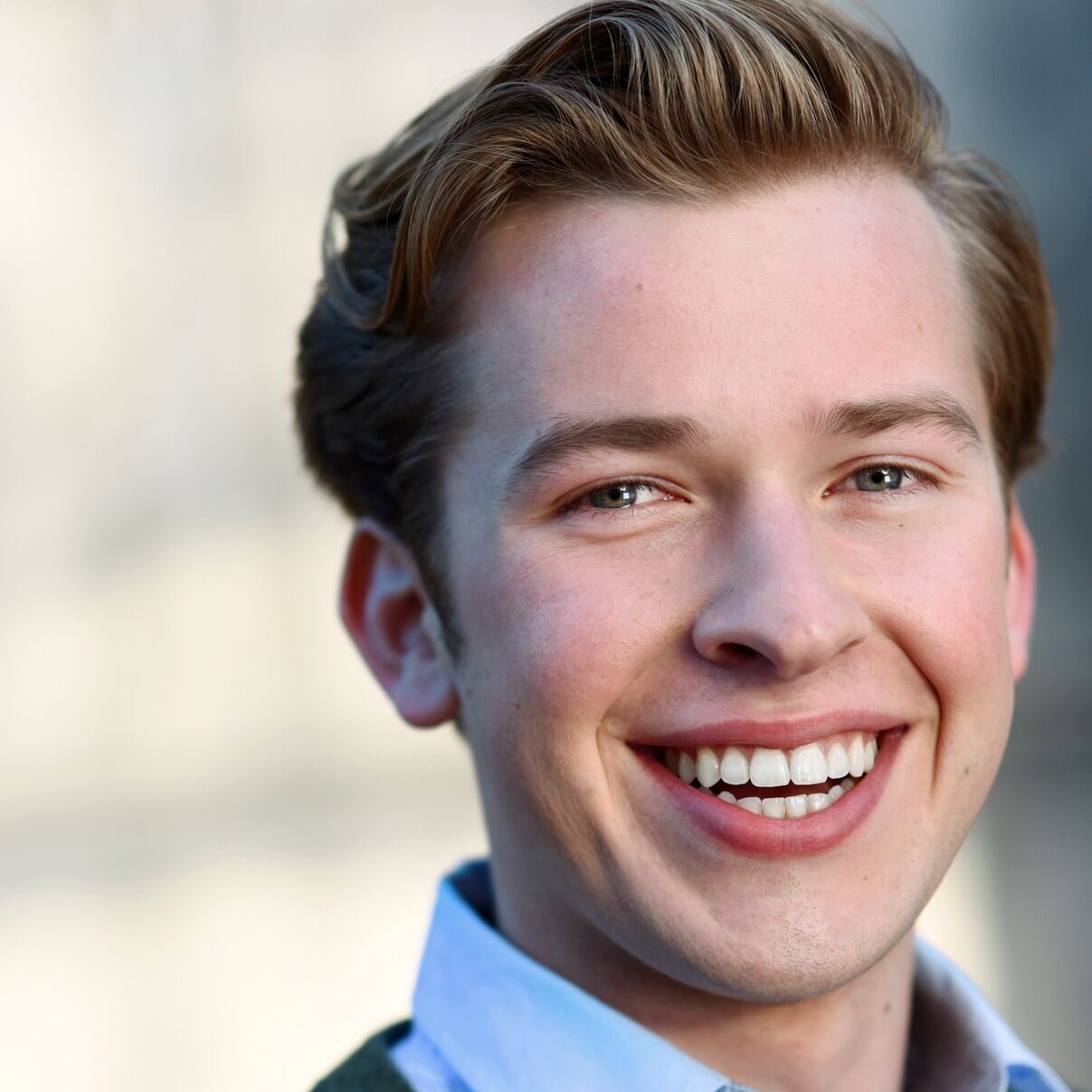
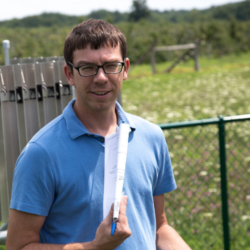
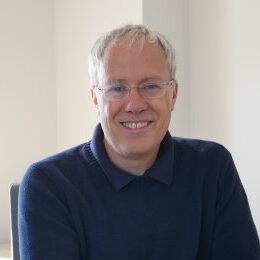
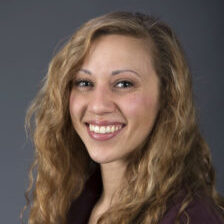
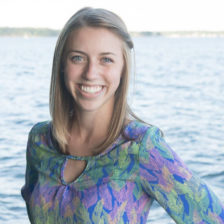
Numerical Weather Prediction (NWP) models play a vital role in forecasting critical weather variables such as temperature, wind, and precipitation. However, these models often exhibit errors and biases due to varying physical processes, spatial resolutions, and regional characteristics. This project aims to quantify and predict NWP errors across the New York State (NYS) and Oklahoma (OK) Mesonets using machine learning (ML) approaches. Our goal is to develop accurate, region-specific ML models that forecast temperature, wind, and precipitation errors, providing user-tailored insights for National Weather Service (NWS) partners.
Our team recentl publishing a peer-reviewed study verifying major NWP models using NYS Mesonet data and developing a Long Short-Term Memory (LSTM) model that successfully predicts forecast errors. To overcome challenges like model variability and regional differences, we are conducting an extensive ML architecture intercomparison and analyzing the relationship between NWP errors and geographic factors (e.g., land-use, topography). We are strengthening partnerships with NWS offices to co-develop practical, user-focused deliverables, ensuring that our ML-driven insights enhance operational weather forecasting and decision-making.
Highlighted Publications and Releases
Gaudet, L. C., K. J. Sulia, R. D. Torn, and N. P. Bassill, 2024: Verification of the Global Forecast System, North American Mesoscale Forecast System, and High-Resolution Rapid Refresh Model Near-Surface Forecasts by Use of the New York State Mesonet. Wea. Forecasting, 39, 369–386, https://doi.org/10.1175/WAF-D-23-0094.1.
Advancing AI in Earth System Science Research
Join us to unlock AI’s potential in science.